Alaya AI is an innovative AI data annotation platform designed to advance the AI industry through blockchain technology, zero-knowledge proofs, a shared economy model, and advanced AI data labeling and organization techniques. This project allows users to contribute data while being rewarded, leveraging blockchain and ZK technology to protect user privacy and data ownership.
Alaya AI collects data through user participation in answering questions and utilizes an integrated AI system to assess the accuracy of user contributions, rewarding them with corresponding token incentives. As users’ NFT levels increase, the difficulty of questions gradually rises, covering various types of questions from general knowledge to specialized fields. Ultimately, Alaya AI standardizes the collected data for various AI models to recognize and train on.
Market Analysis
Traditional economics views labor, land, and capital as the primary factors of production, but in the era of artificial intelligence, the logic may have subtly changed, with algorithms, data, and computing power becoming the new triumvirate of production. For current explorations of large language models, algorithm adjustments are still based on Transformer technology, while computing power continues to increase. However, high-quality data remains the key metric limiting breakthroughs in models and algorithms. As companies begin training their own AI models, the demand for data is skyrocketing.
In the traditional world, the data annotation business has supported a multi-billion-dollar market, with well-known companies including Scale AI, Appen, Lionbridge, and CloudFactory. However, traditional data annotation businesses have struggled to reach a global user base, exacerbating inequality between different regions. Reports indicate that outsourced data annotators in Kenya, utilized by OpenAI, earn less than $1.5 per hour and annotate around 200,000 words per day.
In Web3, utilizing blockchain technology, data ownership can belong to individual data providers. Decentralized data storage and trading mechanisms enable individuals to better control their data assets, facilitating transactions and authorizations on-demand, thereby gaining corresponding incentives and rewards. This model better safeguards the rights of data annotators. With the immutability and traceability features of blockchain, Web3 data services can provide higher transparency and reliability. Every data transaction, annotation task allocation, and completion status will be recorded on the chain, accessible for verification, thereby reducing the possibility of fraud and malfeasance. Data users can trust data on the chain alone, without requiring additional trust endorsements.
Product Design
To lower the barrier to user participation, Alaya AI has designed a gamified product that collects data through user interaction in the form of answering questions while using cryptographic algorithms to ensure user privacy is not compromised.
For AI, By AI. Similar to the concept of reinforcement learning, Alaya AI integrates AI within the product to help identify the quality of data, assess the accuracy of user judgments on AI data, and determine their contribution level, thereby providing incentives accordingly. Additionally, Alaya AI will introduce a reputation mechanism and quality verification nodes to decentralized verification of annotated results. Through random sampling and cross-validation by quality verification nodes, errors or malicious annotations can be identified more efficiently, maintaining high-quality annotated results. In task allocation, Alaya AI employs an AI algorithm-assisted task allocation method, efficiently matching tasks with users. The more high-quality data a user contributes, the higher their NFT level, and the difficulty of questions also increases accordingly. From general common-sense questions to specific fields (such as driving, gaming, film and television), and finally to advanced fields (medical, technology, algorithms), the complexity of questions escalates.
Feasibility Analysis
Although traditional data annotation companies have suspicions of exploiting employees, this greatly aids the company’s profitability. While Web3 data annotation may enhance human welfare in a more equitable manner, would it economically reduce platform revenue? In reality, Alaya AI increases overall utility by adding diversity.
Traditional data annotation methods not only demand high individual workloads but also struggle to ensure sample quality. Due to low annotation rewards, platforms mostly recruit users from developing regions where education levels are generally low, resulting in a lack of diversity in submitted samples. For advanced AI models requiring specialized knowledge, platforms struggle to recruit suitable annotators.
By integrating token/NFT rewards and referral bonuses, Alaya combines social and gaming elements into ordinary data annotation tasks, effectively expanding the community size and improving retention rates through activities such as daily check-ins. While controlling the reward amount per task for individual users, Alaya’s viral referral system allows the earnings of high-quality users to increase infinitely with the expansion of the social network.
Fundamentally, centralized data platforms of the Web2 era heavily rely on a few users providing a large volume of samples continuously, whereas Alaya reduces the quantity of data contributed by individual users while increasing the number of participating users. With lower individual workload, the quality of contributed data significantly improves, and data representativeness is significantly enhanced. With a larger user base reached, decentralized data annotation platforms collect data that more accurately represents the collective intelligence of humanity, eliminating sampling biases.
To prevent individual users’ unfamiliarity with problem domains/maliciously submitted incorrect answers from affecting data quality, the Alaya AI platform adopts a normal distribution model to validate data and automatically exclude or standardize extreme values. Additionally, leveraging proprietary optimization algorithms, Alaya verifies through cross-referencing user answers and weights, eliminating the need for manual inspection and correction, further reducing data costs. The data validity threshold will be dynamically adjusted based on the sample size of each task to avoid overcorrection, minimizing data distortion.
Technical Features
As an intermediary between data producers (individual users) and data consumers (AI models), Alaya AI collects user-annotated data, processes it, and delivers it to AI models.
Alaya AI adopts an innovative Micro Data Model (Tiny Data), optimizing and iterating on traditional big data to enhance deep learning training effects from multiple aspects:
- Data Quality Optimization: The Micro Data Model focuses on high-quality small-scale datasets, improving data accuracy and consistency through data cleaning and labeling optimization. High-quality training data effectively enhances model generalization and robustness, reducing the negative impact of noisy data on model performance.
- Data Feature Compression: The Micro Data Model employs feature engineering and data compression techniques to extract key features and eliminate redundant and irrelevant information. The condensed dataset contains a higher density of useful information, accelerating model convergence speed while reducing computational resource consumption.
- Sample Balance Optimization: The performance of deep learning models is often affected by imbalanced data distributions. The Micro Data Model utilizes intelligent data sampling strategies to balance samples from different categories, ensuring that the model has sufficient training data in each category to improve classification accuracy.
- Active Learning Strategy: The Micro Data Model introduces an active learning strategy to dynamically adjust data selection and annotation processes based on model feedback. Active learning prioritizes annotating samples that have the greatest impact on improving the model, avoiding inefficient repetitive labor and improving data utilization efficiency.
- Incremental Learning Mechanism: The Micro Data Model supports incremental learning, continuously adding new data for training on the existing model, achieving iterative optimization of model performance. Incremental learning enables models to continuously learn and evolve, adapting to changing application scenario requirements.
- Transfer Learning Capability: The Micro Data Model possesses transfer learning capabilities, allowing trained models to be applied to similar new tasks, significantly reducing the data requirements and training time for new tasks. Through knowledge transfer and reuse, the Micro Data Model can achieve good training effects in small sample scenarios.
Additionally, Alaya AI integrates AI training and deployment tools, supporting common deep learning frameworks, enabling various AI models to directly recognize and utilize them, thus reducing the cost of upstream model training. Furthermore, leveraging cryptographic algorithms such as zero-knowledge proofs and access control technologies, Alaya AI fully protects user privacy from infringement throughout the process.
Ecological Development
Currently, Alaya AI supports two major mainnets, Arbitrum and opBnB, and allows registration via email. Its mobile app is already available on Google Play.
From a business perspective, Alaya AI has established stable partnerships with over ten AI technology companies, with the number of collaborations continuing to grow. This enables Alaya to achieve stable cash flow realization, allowing it to consistently provide cash and token rewards to users.
From a consumer standpoint, Alaya AI currently boasts over 400,000 registered users, with over 20,000 daily active users, and facilitates over 1,500 on-chain transactions daily. Additionally, Alaya has established a decentralized autonomous community, which will determine the direction of the product openly, transparently, and democratically.
In the future, Alaya AI aims to further integrate with DePIN, embedding itself into integrated AI smart hardware products (e.g., Rabbit R1), to gather data from users’ daily interactions and utilize idle computing power of devices. Moreover, through collaborations with decentralized computing platforms (such as Akash and Golem), Alaya AI can establish a unified marketplace for AI data and computing power, allowing AI developers to focus solely on algorithm optimization. Regarding data storage, Alaya AI can store annotated data with decentralized storage protocols like IPFS and Arweave, and actively collaborate with decentralized AI model markets (such as Bittensor) to train decentralized models with decentralized data.
Token Incentives
Alaya AI’s token system primarily consists of two parts: user incentives and ecosystem incentives.
The first part is the AIA token, which serves as Alaya’s fundamental platform incentive token. Users receive AIA tokens as rewards for completing tasks, achieving milestones, and participating in other activities within the product. AIA tokens can also be used for upgrading user NFTs, participating in events, and obtaining unique achievements, all of which enhance players’ basic output within the product. AIA tokens possess basic output and consumption scenarios, with both aspects mutually reinforcing each other.
The second part is the AGT token, which serves as Alaya’s governance token, with a maximum issuance of 5 billion. AGT is used for ecosystem development, upgrading advanced NFTs, and participating in community governance activities. Users must hold AGT to participate in community governance, data review, and request issuance.
Alaya AI’s dual-token model separates economic incentives from governance, thereby avoiding significant fluctuations in governance tokens that may affect the stability of the system’s economic incentives. This enhances the overall scalability of the system and is more conducive to its long-term benign development.
Competitive Analysis
A comparison of existing decentralized data labeling projects is provided below:
From the competitive analysis perspective, newer projects are likely to perform better in terms of token performance compared to older ones. Additionally, projects with real user data support significantly outperform those lacking users. As an emerging project, Alaya AI, with over 400,000 registered users, over 20,000 daily active users, and over 1500 on-chain transactions daily, is likely to receive better value support after its token issuance.
Reference:
Website: https://www.aialaya.io/
Twitter: https://twitter.com/Alaya_AI
Telegram: https://t.me/Alaya_AI
Medium: https://medium.com/@alaya-ai
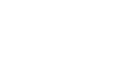